MATLAB、Python、Scilab、Julia比較ページはこちら
https://www.simulationroom999.com/blog/comparison-of-matlab-python-scilab/
はじめに
の、
MATLAB,Python,Scilab,Julia比較 第3章 その114【射影変換、アフィン変換合成⑥】
を書き直したもの。
射影変換とアフィン変換の合成をプログラムで実現する。
今回はPython。
【再掲】数式とパラメータ
まずは、数式とパラメータを再掲。
射影(逆)変換
\(
\begin{eqnarray}
\begin{bmatrix}
x\prime\\
y\prime\\
1
\end{bmatrix}&=&
\begin{bmatrix}
a&b&c\\
d&e&f\\
g&h&1
\end{bmatrix}^{-1}
\begin{bmatrix}
S_x&0&0\\
0&S_y&0\\
0&0&1
\end{bmatrix}^{-1}\\
&&\begin{bmatrix}
1&0&T_x\\
0&1&T_y\\
0&0&1
\end{bmatrix}^{-1}
\begin{bmatrix}
\cos(\theta)&-\sin(\theta)&0\\
\sin(\theta)&\cos(\theta)&0\\
0&0&1
\end{bmatrix}^{-1}
\begin{bmatrix}
x\\
y\\
1
\end{bmatrix}
\end{eqnarray}
\)
パラメータ
射影変換
\(
\begin{eqnarray}
(-1,-1)→&(-0.5,-0.8)\\
(-1,1)→&(-0.8,0.8)\\
(1,1)→&(1,1)\\
(1,-1)→&(0.4,-1)\\
\end{eqnarray}
\)
伸縮アフィン
縦横を1/2へ縮小
移動アフィン
x軸方向+0.5
回転アフィン
+60°
これをPython(NumPy)で実現する。
Pythonコード
Pythonコードは以下。
import numpy as np
import cv2
# 射影変換関数
def homograpy(img, matrix):
# 画像サイズ取得
hight, width = img.shape
# 中心を0とした座標系を生成
x_axis = np.linspace(-1, 1, width);
y_axis = np.linspace(-1, 1, hight);
xim,yim = np.meshgrid(x_axis, y_axis);
# 座標x,y,1の3次元ベクトルの配列
# reshapeで行ベクトル化、「*」で式展開
points=np.array([[*xim.reshape(width*hight)],
[*yim.reshape(width*hight)],
[*np.ones((width*hight))]])
# 変換元座標算出
points_homography = matrix @ points;
# 画像と同一形状の2次元配列に変換元座標配列を生成
dx = points_homography[0,:].reshape(hight,width)
dy = points_homography[1,:].reshape(hight,width)
ds = points_homography[2,:].reshape(hight,width)
dx = dx/ds
dy = dy/ds
# 変換元座標をピクセル位置に変換
v = np.clip((dx + 1) * width / 2, 0, width-1).astype('i')
h = np.clip((dy + 1) * hight / 2, 0, hight-1).astype('i')
# 元画像と変換元座標を元に変換先へコピー
return img[h, v]
# キャンパス拡張
def canvas_expansion(img, x, y):
H,W=img.shape
WID=W+x
HID=H+y
e_img = np.zeros((HID, WID),dtype='uint8')
e_img[int((HID-H)/2):int((HID+H)/2), int((WID-W)/2):int((WID+W)/2)] = img;
img = e_img
return img
def homography_affine():
# 入力画像の読み込み
img = cv2.imread("dog.jpg")
b = img[:,:,0]
g = img[:,:,1]
r = img[:,:,2]
# SDTVグレースケール
img = np.array(0.2990 * r + 0.5870 * g + 0.1140 * b, dtype='uint8')
# キャンパス拡張
img = canvas_expansion(img, 100, 100)
sx = 0.5;
sy = 0.5;
tx = 0.5;
ty = 0;
theta = 60/180*np.pi;
x0=-1; y0=-1; # 左上
x1=-1; y1= 1; # 左下
x2= 1; y2= 1; # 右下
x3= 1; y3=-1; # 右上
x0t=-0.5; y0t=-0.8; # 左上変換先
x1t=-0.8; y1t= 0.8; # 左下変換先
x2t= 1; y2t= 1; # 右下変換先
x3t= 0.4; y3t=-1; # 右上変換先
mat = np.array([ [x0, y0, 1, 0, 0, 0, -x0*x0t, -y0*x0t],
[ 0, 0, 0, x0, y0, 1, -x0*y0t, -y0*y0t],
[x1, y1, 1, 0, 0, 0, -x1*x1t, -y1*x1t],
[ 0, 0, 0, x1, y1, 1, -x1*y1t, -y1*y1t],
[x2, y2, 1, 0, 0, 0, -x2*x2t, -y2*x2t],
[ 0, 0, 0, x2, y2, 1, -x2*y2t, -y2*y2t],
[x3, y3, 1, 0, 0, 0, -x3*x3t, -y3*x3t],
[ 0, 0, 0, x3, y3, 1, -x3*y3t, -y3*y3t]])
dst = np.array([x0t, y0t, x1t, y1t, x2t, y2t, x3t, y3t]).T
res = np.linalg.inv(mat)@dst;
homo_matrix = np.array([ [res[0], res[1], res[2]],
[res[3], res[4], res[5]],
[res[6], res[7], 1 ]])
homo_matrix = np.linalg.inv(homo_matrix)
scaling_matrix = np.array([[ sx, 0, 0],
[ 0, sy, 0],
[ 0, 0, 1]])
scaling_matrix = np.linalg.inv(scaling_matrix)
translation_matrix = np.array([[ 1, 0, tx],
[ 0, 1, -ty],
[ 0, 0, 1]]);
translation_matrix = np.linalg.inv(translation_matrix)
rotation_matrix = np.array([[ np.cos(theta), -np.sin(theta), 0],
[ np.sin(theta), np.cos(theta), 0],
[ 0, 0, 1]]);
matrix = homo_matrix@scaling_matrix@translation_matrix@rotation_matrix;
# 射影変換
homography_img = homograpy(img, matrix)
# グレースケール画像の書き込み
cv2.imwrite("dog_homography_affine.jpg", homography_img)
return;
homography_affine()
処理結果
処理結果は以下。
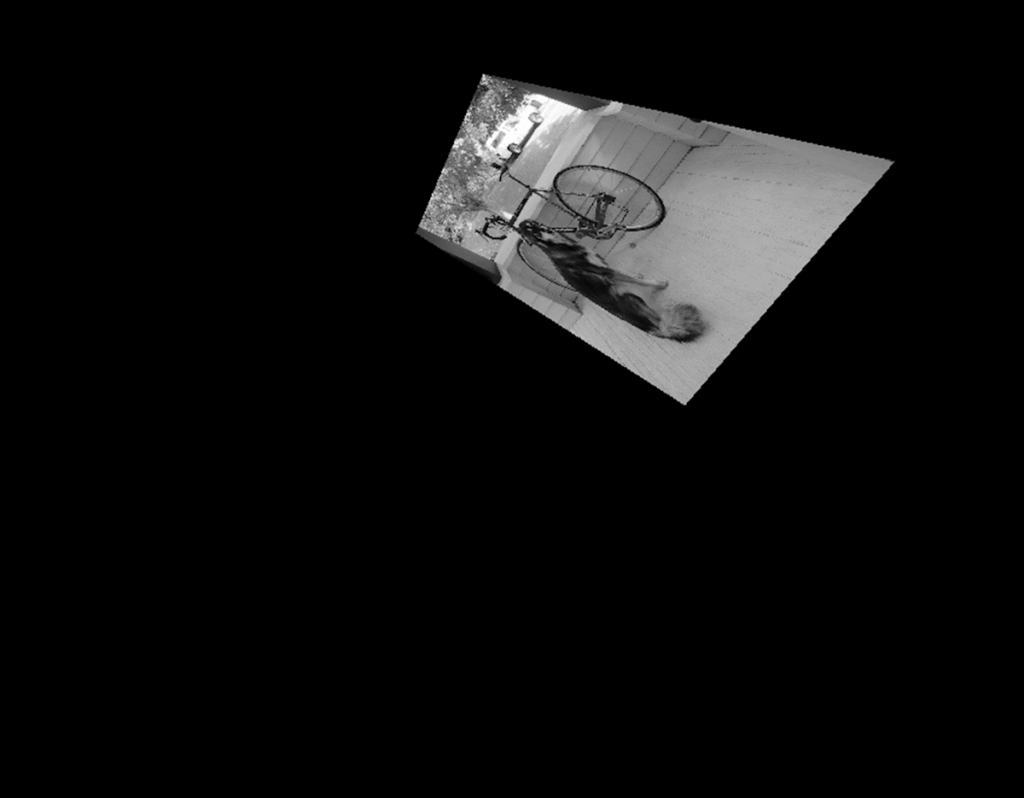
考察
考察
これも、処理の流れとしてはMATLABと一緒。
canvas_expansionとhomographyの関数は今までのものと変更なし。
変わったのはパラメータの部分だけ。
射影変換にアフィン変換行列を渡せば、アフィン変換ができることを知っていれば、問題無いということになる。
まとめ
- 射影変換とアフィン変換の合成をPython(NumPy)で実施。
- 問題無く動作。
- 射影変換にアフィン変換行列を渡せば、アフィン変換ができることを知っていればOK。
MATLAB、Python、Scilab、Julia比較ページはこちら
コメント